Australian merchant payment platform fintech provider, mx51, is utilizing Snowflake to support the company’s analytics and customer service activities.
Snowflake, the Data Cloud company, has announced it has been selected by merchant payment platform fintech provider, mx51, to support the company’s analytics and customer service activities.
Australian-based mx51 provides a suite of white-label services to banks and finance institutions which allows them to offer in-store, phone and online payment capabilities to their customers.
Steven Hadley, Chief Product Officer at mx51, said when the company was first established its small team of staff relied on little more than a series of relational databases to store data generated by its microservices.
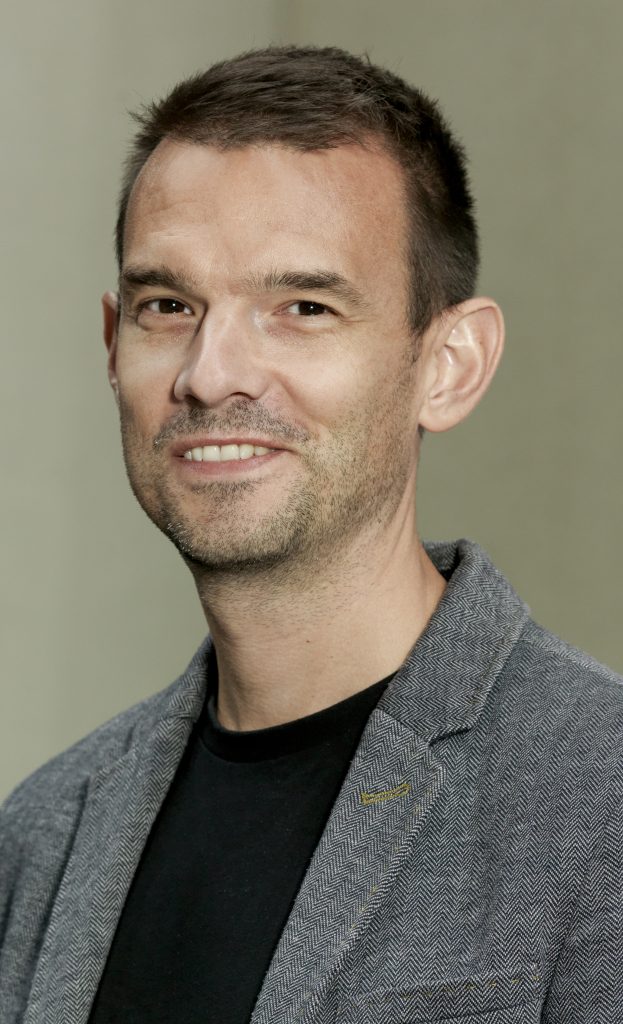
“Back then, a key driver for us was wanting to have a better ability to run queries,” he said. “Prior to Snowflake, if you wanted to run queries across multiple databases there was no easy way for this to be done.”
The hunt for an alternative
The mx51 IT team began by evaluating a range of alternatives for a new data platform. Hosting an on-premise instance of a Postgres database was also considered, however the management and maintenance overheads were deemed to be too high.
“At the end of the day, it became clear that Snowflake was the best fit for our requirements,” said Hadley. “We were particularly impressed with its ability to scale and to integrate with our front-end tools.”
Deployment began with an initial proof-of-concept. This involved extracting data from a number of Postgres databases and providing answers to relatively simple user queries. Once it was clear that the approach worked, additional data was added and user numbers quickly grew.
“Snowflake now underpins our entire business intelligence efforts,” said Hadley “It is used by everyone from our product team to finance and senior management who use it to assist in strategic planning.
“It allows queries to be quickly answered and provides a clear picture of what is going on within the payments infrastructures of our customers.”
Improved customer support
As well as providing in-house support for analytics and business intelligence activity, Snowflake is also used by the mx51 support team to resolve any merchant issues that might arise. For example, if a retailer is experiencing issues with a terminal, data from the machine can be quickly accessed and analyzed to determine the best resolution.
“It also allows us to provide proactive support for our customer’s customers,” said Hadley. “If a problem is identified that may have an impact on other merchants, our team can contact them and make them aware that a solution is being devised ahead of potential issues occurring. This sets us apart from other payment firms on the market.”
Future plans
With growth in merchant and transaction numbers continuing to increase, Hadley said there will be further ways in which Snowflake can support the company in the future.
“We will be using Snowflake to support more detailed analysis and reporting of transactions for merchants,” he said. “This will allow merchants to do things such as altering their trading patterns or augmenting their product ranges to improve sales and revenues.”
This will be achieved by enhancing a merchant-facing portal that provides a data feed from installed terminals. The dashboard will give a holistic view of the merchant’s payment system, as well as providing the ability to drill down to see specific transactions.
“I’m confident we now have in place a data platform that will be able to grow and support us for many years to come,” said Hadley. “It will also enable us to gain insights and use BI tools in ways that previously would not have been possible.”
We asked Mike Gouline, Data Architect, mx51, further questions to find out more.
Why were you impressed with Snowflake’s ability to scale?
Snowflake allows us to scale the compute horizontally and vertically, which means that whether we need more horsepower for complex queries or just the ability to handle more queries concurrently, there are cost-effective options to achieve that. This is very important for our white label model of operation, because no two tenants are the same in their volumes, features and expectations.
Can you explain how Snowflake successfully integrated with your front-end tools?
Snowflake is widely supported in the data ecosystem, so every business intelligence and visualisation tool that we currently use, including Metabase and Power BI, and even tools that we evaluated throughout our data journey had first-party support for Snowflake. The same goes for integration and workflow tools we use to load, unload and transform data in Snowflake, to surface insights in our customer-facing dashboards.
How efficient did you find the deployment process overall?
Ease of deployment is crucial for our operations, because all infrastructure has to be provisioned separately for each tenant. As a result, we are very selective about the services we use outside of the main cloud provider, to avoid complicating the automation process. However, Snowflake provides an admin panel to self-serve creating new accounts and thanks to an open-source Terraform provider, we can automate all configuration and security settings through the same means as all the other AWS infrastructure without resorting to many manual steps.
Can you explain how Snowflake allows queries to be quickly answered?
Answering queries, whether they are business questions answered via visualisation tools (e.g. Metabase or Power BI) or low-level queries surfaced via the customer-facing dashboards, the biggest challenge is getting all the data from disparate sources in one place and up to date ready to be combined, joined and aggregated any way the task requires. This part of the data workflow is aided by Snowflake’s loading features, such as Snowpipe, streams and tasks, which enable us to easily load anything we extract from the relational databases at scale.
Once everything is in Snowflake, in semi-structured or structured form, scalable compute warehouses help run queries as quickly as we need and in parallel, meaning that a sudden spike in data analytics activity before a board meeting has no impact on any production processes in the background. Not having to wait a long time for results also means that users are encouraged to ask more complex questions of the data, instead of having to make do with basic summaries if digging deeper means waiting 10 minutes for each result.
When you have thousands of payment terminals generating millions of diagnostic events, trying to find out what happened to a particular one on a particular afternoon can be daunting. Snowflake’s performance helps us run very complex SQL queries, sometimes sourced from domain experts in engineering, to help the support team quickly resolve issues for our merchants.
How does Snowflake provide a clear picture of what is going on within the payments infrastructures of your customers?
Every piece of data about the tenant’s infrastructure already is or can be ingested into Snowflake. This means that whenever there is an active issue with one of the merchant’s terminals, or we want to check the impact of a particular future change, all we have to do is log into a BI tool and run a query.
For example, when the support team identifies a particular problematic payment terminal configuration after troubleshooting on the phone with a merchant, they can immediately check how many other merchants have this configuration and put together a plan with engineering to fix it behind the scenes or proactively contact the businesses with a fix.
Moving beyond descriptive and diagnostic analytics, Snowflake allows us to seamlessly transition into the predictive and prescriptive realm, running ML-based anomaly detection and feeding the alerts back to the customer-facing dashboards well before human users identify the problem, or clustering merchants based on their usage patterns to recommend the tenant’s relevant features and products to them.
Why did you choose to use Snowflake?
There is no shortage of data products on the market. Our aim is to keep the product offering as cloud agnostic as possible, so we wanted to store the data somewhere that works on all three major clouds: AWS, Azure and Google Cloud.
Similarly, we try to avoid deep vendor lock-in when it comes to consuming the data. Part of it is the front-end tool support discussed earlier, but it’s also about the amount of training and effort required for new team members to become familiar with querying the data. Snowflake is based on SQL, which means that anyone with some experience in other relational databases can start using it within minutes or hours, not days or weeks. We have team members with no engineering or scientific background happily writing SQL queries to answer their own questions.