Ben Bromhead, New Zealand-based Chief Technology Officer at Instaclustr by NetApp, takes a look at three trends poised to reshape many more CIOs’ enterprise analytics practices throughout the second half of 2022 and beyond.
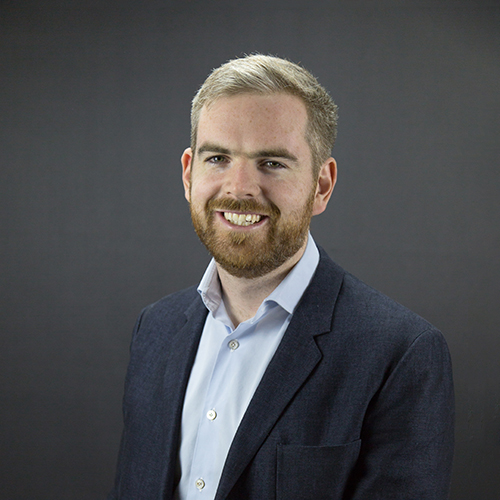
Enterprise technology leaders may well look back upon 2020 to 2022 as especially pivotal years for analytics technologies and practices. A growing number of CIOs are championing transformative analytics capabilities within their organizations that will likely shape prevailing strategies for years to follow.
But for those who haven’t, it’s certainly not too late.
Three key trends have been coming to fruition this year. Taken together, they are contributing to a sea change in analytics strategy and execution. This is the year that tapping into Machine Learning (ML) for predictive analytics within the database has shifted categories, from ‘emerging tech’ to an enterprise-grade approach that is ready for prime time.
Second, ‘data mesh’ strategies are also proving ready to unlock the full potential of data lakes across enterprise teams. Third, open source communities in the data and analytics space are similarly flexing newfound strengths – emphasizing both the immediate and long-term value of utilizing fully open source technologies in this space.
Let’s take a closer look at these three trends poised to reshape many more CIOs’ enterprise analytics practices throughout the second half of 2022 and beyond.
Enterprise database management systems are benefiting from predictive analytics, powered by Machine Learning
It’s no secret that technology leaders have faced challenging limitations addressing the complexity of database management. Utilizing traditional methods, efficient query design requires overcoming an unfathomable range of potential data designs, anticipating data usage patterns that are beyond prediction, and addressing data storage management outside of even the database’s purview.
It can be a mess, and even a CIO’s most experienced database administrators struggle to achieve optimized queries under these conditions. They do their best to harness the traditional data traffic pattern and storage growth analytics available, but there’s clearly been room for improvement.
Machine Learning has proven to have a transformative impact in some areas, whereas others have failed to really demonstrate value. In the past, I’ve talked about the potential for ML and AI to have an impact on query speed/index creation and general database performance.
These solutions offer the capabilities to predict the location of data with a high degree of accuracy, and then create efficient data indexes and optimize storage management automatically. Unfortunately, while we are still seeing some very interesting work come out of academia related to this, there is no real commercial or practical adoption outside of some Edge cases.
I’m still bullish on this use case as it’s a great problem domain for Machine Learning (well-defined scope, measurable outcomes, easy to train), and while there will be some CIOs who take a ‘second to adopt’ mentality, others who lean into it early will reap the rewards.
The distributed ‘data mesh’ approach to realizing data lakes’ full potential is winning mainstream enterprise adoption
CIOs are increasingly taking advantage of data lake implementations that deliver better data visibility and more effective analytics capabilities. That said, data integrations that allow increased data utilization are essential to realizing the true potential of data lakes and to empowering CIOs’ teams to achieve the timely data analysis required.
Enterprises are adopting solutions such as open source Apache Kafka in order to harness data and utilize transactional production workloads in real-time within data lakes. They’re also increasingly using Kafka Connect to operate analytics services that need both data lake connectivity and active data awareness.
‘Data mesh’ strategies now give individual teams the direct control to access, curate and manage the data most important to their activities. This practice – which brings data management in line with the same modernized concepts behind distributed architecture – allows teams to optimize analytics via their own decentralized self-service command over data. Getting this right drives superior results and has caused CIOs across industries to take notice.
Open source communities are proving who really controls essential data and analytics technologies
Today, vendors that choose to shift their open source solutions to proprietary licensing are increasingly met with immediate reprisals. Project communities now have the power to lead and support high-quality open source forks that render those original vendors unnecessary and irrelevant.
A stark example was recently provided by Elasticsearch, where the vendor introduced a more proprietary license that swiftly precipitated the community’s introduction of OpenSearch.
I expect this trend will only intensify moving forward, driving savvy CIOs and other enterprise technology leaders to embrace open source data and analytics tooling wherever possible. (And also: what CIO isn’t under increasing budget constraints right now? The cost and flexibility advantages of using fully open source data and analytics technologies are only going to get more appealing-and CIOs can adopt them without sacrificing performance or features.)
An important crossroads in analytics strategy
CIOs stand at a rare and pivotal moment of opportunity right now. Leaders can now position their enterprises to achieve significantly more rapid and efficient insights, by anticipating and embracing strategies around analytics technology trends that will be synonymous with enterprise success for years to come.