Artificial Intelligence is a force multiplier for stretched IT Ops teams, but Rafi Katanasho, APAC Chief Technology Officer and Solution Sales Vice President, Dynatrace, says the results it produces could be made more precise by utilising a different model.
The trend towards AI augmentation in many technology and business domains shows no signs of a slowdown. That’s in part because the drivers for using AI are becoming more pronounced over time.
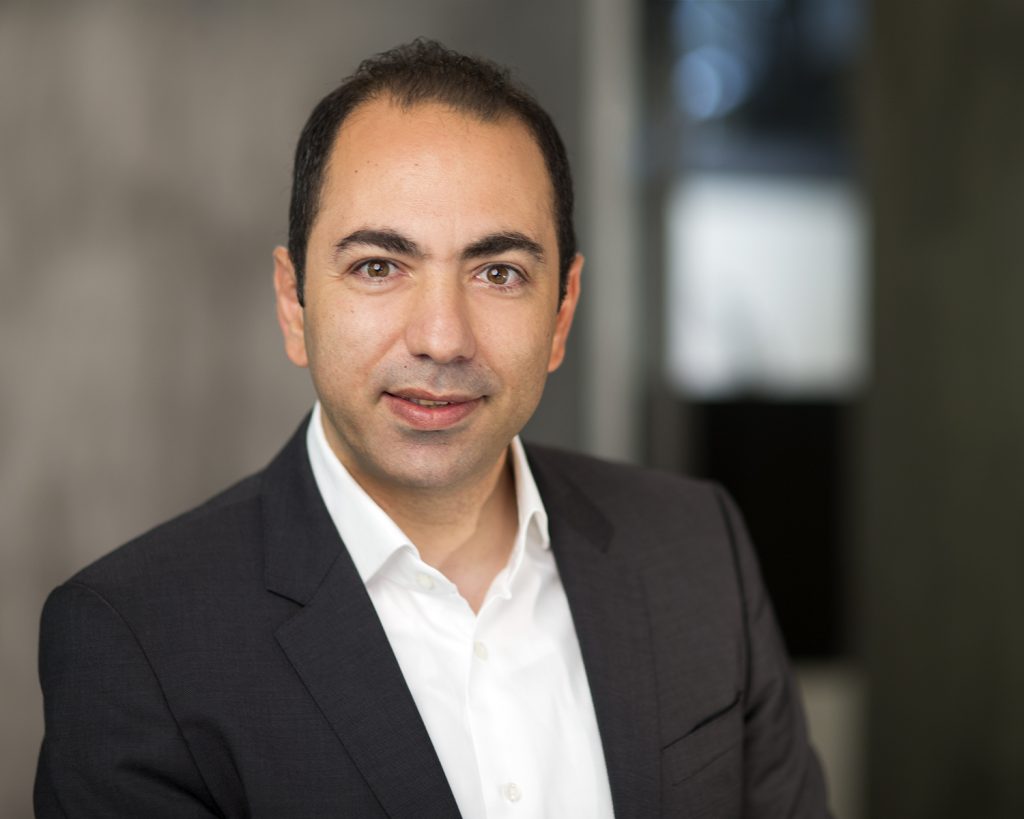
Augmenting IT operations with AI – AIOps – was positioned from day one as a way to help teams maintain oversight and control over highly complex and constantly changing environments.
If anything, the pace of change in IT environments is now faster – recent research shows Digital Transformation accelerated for 90% of organizations in the past year alone.
The complexity of modern environments was already judged as being beyond human capabilities alone in 2020. Add another three years of change and it’s perhaps no surprise to see organizations leaning even more heavily on AI to bridge operational gaps while enabling the current pace of innovation to proceed.
What this is driving, however, is a review of the AI – particularly Machine Learning – algorithms in use, particularly the extent to which they’re able to create the accurate insights needed to reduce time and effort of operations teams in diagnosing and remediating issues or performance bottlenecks.
As more organizations embed AI into their IT operations, many have become aware of a fundamental limitation to some AIOps approaches. This limitation concerns the use of correlation-based Machine Learning models over causal correlation analysis models.
This distinction may not be well understood but is important for teams looking to get the most benefit out of AIOps.
Correlation versus causation
Correlation-based Machine Learning algorithms are traditionally built on the assumption that the future will look a lot like the past.
Applications and servers produce logs, metrics, traces and other telemetry data. This is fed into a Machine Learning algorithm, which parses the data and suggests potential root causes for the problem based on previous observations that exhibited similar disruptive conditions or behaviours. While alerting teams to errors, a human must then investigate what caused those errors in the first place.
This approach can work well in a largely static environment, but these are becoming the exception rather than the rule.
Most environments today are cloud-based, constantly scaling up or down as business needs change, or spinning up and tearing down resources to truly only pay for what they need.
This kind of dynamism does not lend itself to a correlation-based approach, because the algorithm is unable to produce accurate and actionable results fast enough. Additionally, every change requires the correlation-based Machine Learning model to relearn how the environment works.
An inability to handle novel situations is a significant liability; the Machine Learning model, in this instance, becomes less of an augmentation and force multiplier and more of a burden on the teams it is meant to be assisting.
The alternative approach to consider is one that uses causal-based correlation, an approach that can be simplified as ‘causal AI’.
Causal AI is considered a better fit for highly dynamic environments. It is able to continuously monitor the entire system and application elements and maps everything in real-time, including relationships. With this real-time and comprehensive knowledge, causal AI can determine the exact issue, where to find it and how to fix it. This eliminates complexity for operations teams and drives faster mean-time-to-resolution (MTTR).
How Causal AI produces better results
Causal AI derives its advantages in part due to the fault tree approach it takes. This is best illustrated with an example.
Consider an application that is performing slowly in receiving search requests. A fault analysis using a causal AI model would begin by first looking at the starting node of the tree (the application) before digging into the application’s dependencies, which could include third-party API calls or use of external code libraries. The process continues down the ‘tree’, testing each branch and twig for anomalies until the system identifies the root cause of the problem.
This approach separates causal AI from purely correlation-based models. It moves beyond statistical convergence and beneath surface-level correlation between variables.
It instead produces a deeper and near real-time understanding of the true cause-and-effect relationships in the data. That means more precise answers for IT Ops teams and an ability to rapidly trace problems back to their root causes.
It should also lead to more continuous improvements to the environment; first, because causal AI models help teams better understand how to reconfigure and avoid similar disruptions in the future; and second, by its ability to model and simulate futures that aren’t mapped out by past events.
Using virtual experiments, causal AI can answer conceptual and counterfactual questions to model a wide range of potential outcomes. This form of predictive analytics enables organizations to anticipate situations and prepare contingencies.