João Paulo Tavares, Leader of Solutions Architecture and Pre-Sales, Semantix, explains how focusing on information quality significantly improves data management results.
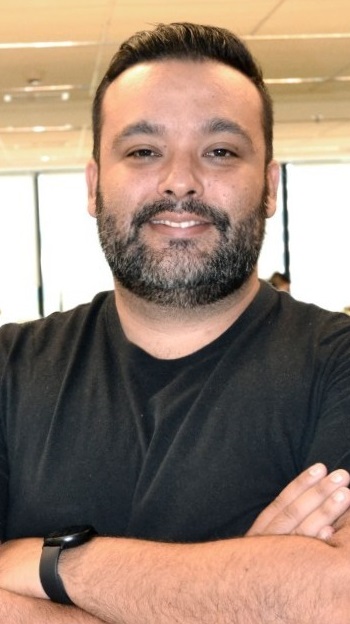
Often defined as the ‘little brother of Big Data’, Small Data is a set of accessible, informative and actionable data that is easy to understand without the need for complex systems and machines. But what is the impact of this on business data analysis?
Small Data is the strategy that focuses on the quality of the collected information, not the volume. The goal is to have only relevant information for actions and campaigns. It is because there are already many tools on the market capable of handling large volumes of data, either by collecting, storing or interpreting it, and much of this data may not be usable for decision-making. In this sense, a broader view of market data is crucial, and Small Data can filter this data precisely.
Common examples of Small Data include:
- Customer Relationship Management (CRM) and Enterprise Resource Planning (ERP) system data
- Marketing material purchase information, raw materials and equipment
- Product and customer sales information
- Customer behavior data
- Online shopping cart data
- Customer satisfaction surveys
- Individual interviews
What are the differences between Small and Big Data?
Data collected through Big Data comes from different sources, external or internal, while Small Data comes from sources within the company itself. Small data is typically included in transaction processing systems and collected before being added to the database or cache layer. If instant analytical queries are needed, databases will have read replicas.
Data Processing: As transaction systems create most of the Small Data, the analyses are usually batch oriented. Only in exceptional cases are queries run directly on transaction systems.
Scalability: Small Data systems often scale vertically. This scaling increases capacity by adding more resources to the same machine. Vertical scaling is more expensive but simpler to manage.
Data Science: Machine Learning algorithms require properly encoded and well-structured input data, especially regarding input data from transactional systems such as a data warehouse or data lake. Because the data preparation stage is limited, machine learning algorithms using Small Data will be easier to implement.
Data Security: The security of small data fragments, which reside in transactional systems or corporate data storage, includes features such as data encryption, user privileges, and more. The corresponding database vendors provide these security features.
Why are companies focusing more on this type of data?
Small Data is more human-centric and can be leveraged effectively in crucial decision-making situations. Data analysis, even when related to Artificial Intelligence (AI) development, should be based on recently obtained and smaller quantities of data. Additionally, large-scale data collection, typically associated with Big Data approaches (including gathering large amounts of data for analytical purposes), poses a challenge for many organizations.
Even if Big Data is available, the costs, time and energy required to implement conventional supervised machine learning can still be challenging. Furthermore, decision-making by humans and AI has become more complex and demanding, requiring a greater variety of data for better situational awareness.
Real-time Information
Small Data is always available, allowing for quick or even real-time decision-making. These are some advisable actions based on this strategy:
- Understanding the triggers that make customers purchase
- Improving lead generation processes
- Changing how you market your products
- Adjusting marketing strategies in real-time
Small Data has also proven its effectiveness in addressing similar applications for problem-solving and decision-making. And the fact that it is more specific and manageable makes it an excellent complement to a wide variety of information – including insights from Big Data.